Machine Learning article
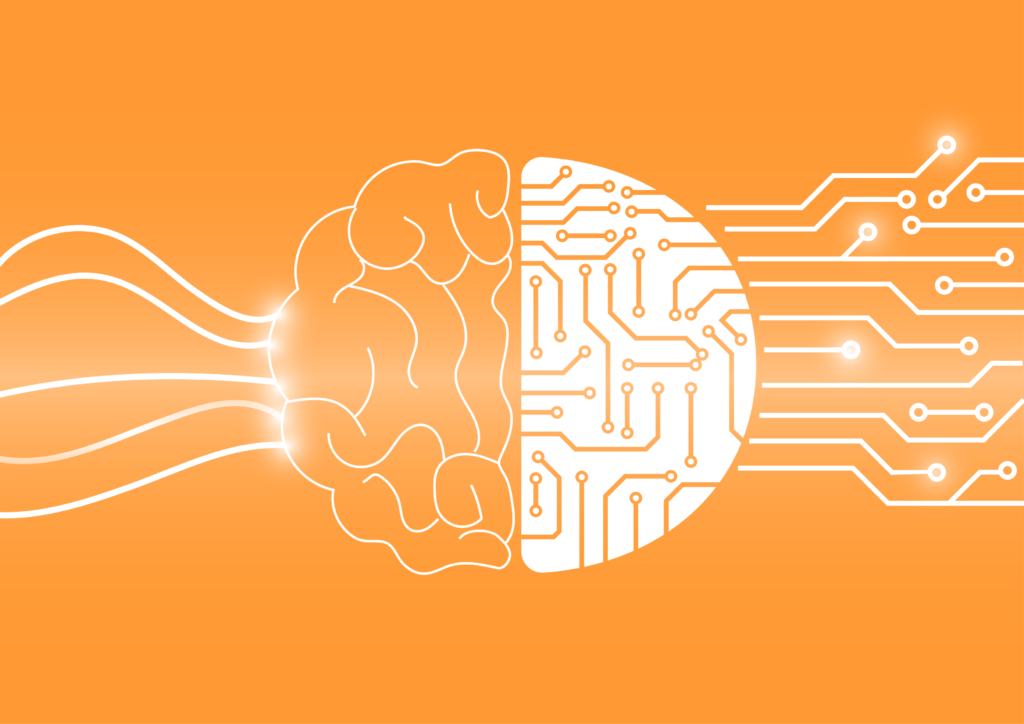
Over the past few years, Machine Learning in Mining was billed as a magic wand that could make all the problems at the mine and the mill magically disappear. Fast forward to 2019, Machine Learning has now evolved from a set of abstract ideas and hypothetical applications that can be applied at both the mine and mill sides to concrete real world, number crunching, learning models that have proven to increase recovery rates at process plants, increase mining intensity, and reduce MTBF, amongst other applications.
Mining is a dynamic and fluctuating business rife with uncertainties related to resource price, volatile resource fields and large projects that need to be handled throughout their life cycles.
The current process of mineral exploration generated an enormous amount of data in the form of soil samples, chip samples, geochemistry, drill results and test results. Each drill hole represents a tiny snapshot of the processes that make up the earth. A single drill hole can generate 200 megabytes of data and an exploration project can produce terabytes of data if there are more drill holes amongst other types of information.
Machine learning has helped mining companies discover minerals to extract. There are several major companies in the mining domain working on this. As a case in point, Goldspot Discoveries uses machine learning to make finding gold more of a science than art. Earth AI is helping mine explorers identify potentially promising areas. They do so by analyzing data from multiple sources and using a machine learning algorithm to identify areas where minerals are likely to be found. Machine learning analyzes 47 layers of remote sensing and geophysical data at once – they can highlight metal ore bodies and alteration haloes as well as map out hard rocks and regolith with extreme detail.
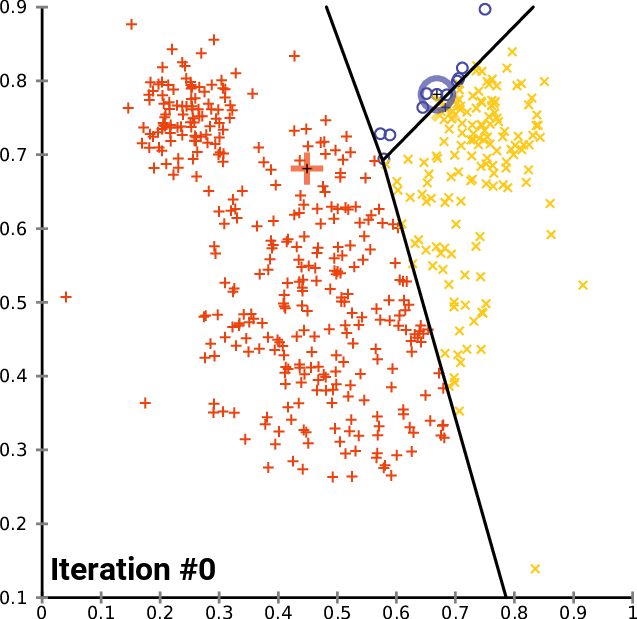
On the Health & Safety side, Machine Learning has helped in reducing accidents and injuries at work. Once enough high quality data is collected from workplace exams, equipment pre-ops, incident reports, near-misses, root causes analyses, corrective actions issued, etc, Machine Learning algorithms can then predict potential failures that not only impact production but are also harmful or fatal to workers, can be predicted. In addition, machine learning has been be used to predict other potential hazards by analyzing patterns in events. Mine Safety uses this information to proactively design engineering and administrative controls to ensure safe mine operations.
Operations teams use this information to plan shifts, miner activities, equipment utilization and asset performance management, as these vary greatly depending on where the mine is located and the mining methods used.
The following are additional advantages of using machine learning in mining:
Accelerating Exploration: Real-time data aids in accelerating timelines for multiple mining stages and decision-making intelligence. Remote sensing data is used for rock-face identification and soil classification, while satellite imagery, aerial photography, geophysical maps, and drone-based monitoring are used to predict mineralization, or the locations of potential ore bodies.
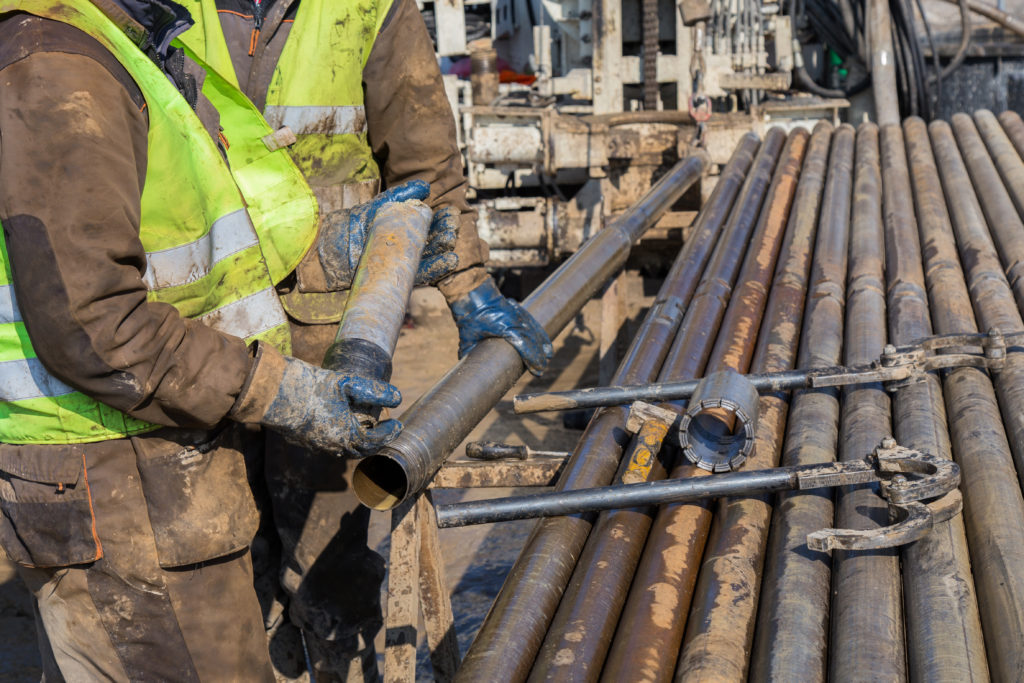
Sorting minerals: Separating useless rocks and debris to get the correct mining material is an expensive endeavor. Some companies have begun to use smart sorting machines that can sort the mined material based on whatever criteria a company wants. This leads to savings in fuel and energy during processing.
Environmental Monitoring: Tracking systems and devices with wireless communications can monitor ecological parameters such as ground water, temperature, and subterranean ventilation changes to assess the impact of mining activities. Technologies such as satellite imagery helps to monitor environmental changes and also predict changes in erosion, wildlife habitats and vegetation.
Predictive Maintenance: With the help of sensors, mining equipment can be monitored and maintained before breakdowns occur. Sensors can monitor temperature, speed, and vibration on machines to take action transforming preventative maintenance into predictive maintenance. By assessing real-time data and analytics, mining operations can be safer for all involved.
Managing Autonomous Equipment: Robotic devices can perform a wide range of mining tasks, including drilling, blasting, loading, hauling, ore sampling, and rescuing trapped miners. This also allows operators to control a drill from a remote location without entering hazardous areas.
Creating Shift Schedules: Performance data about production rates of various activities such as drilling, mucking, shoveling, hauling, bolting and other production and support can be used to create shift wise, daily and weekly schedules to maximize mining intensity, safely.
Balancing Crew Compositions and Rotations: Every miner’s performance is unique to the type of activity they are performing, the type of equipment they are using and their experience. Once enough data is gathered, Machine Learning algorithms can be applied to create crew rosters that optimize for mining intensity, efficiency and safety.
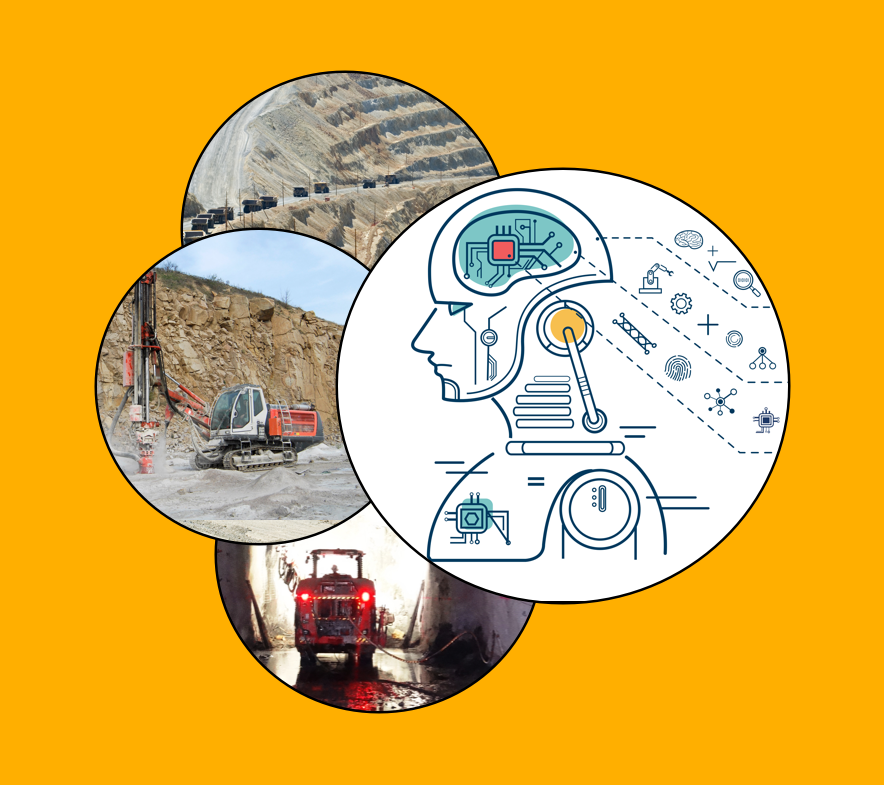
Goldcorp recently partnered with IBM to put their smart technology towards exploration. IBM Watson services are being used to analyze drilling reports, geological survey data and more in determining which areas to explore and to swiftly locate high-value targets. Tomra has developed mineral and ore sorting equipment which uses sensors to separate valuable mineral ores from waste rock.
Mining involves producing commodities, therefore staying competitive in this sector generally implies producing faster and cheaper. When tons of material is being processed in an hour, any small improvement in downtime, processing, or labor costs leads to significant savings.
Mining companies are hyper focused on ways to improve productivity and efficiency. So it is not surprising that some mining companies have been very aggressive about the use of machine learning and autonomous equipment to find ways to improve the same. Mining is unique in a way that it requires huge capital investments and the most common way to be competitive is a ruthless focus on efficiency. This is always going to be important when it comes to making equipment smarter.
With the huge volumes of data generated by any single mine site, machine learning can now be generated to optimize production workflows, operation efficiency and not to mention mine safety. The applications of machine learning will inevitably begin to play a major part in the way mines make decisions and the importance of good data will be key to machine learning cementing its place in the mining industry.
Machine Learning and AI are already having profound impact on the bottom line of mining firms such as Rio Tinto, BHP, Barrick and Freeport McMoran – all early movers into the field, with companies seeing improvements in tons per operator hour, tons per equipment hour, production rates, recovery rates, and availability and utilization rates. There’s no blanket Machine Learning Algorithm or tool and no single metric that will improve once Machine Learning is implemented. Everything starts with the metric the mine is trying to improve or the use case the operation is trying to solve.
While Machine Learning along with AI can be a game-changer that takes your mining operation to the next level, taking your first steps with machine learning does not have to be a monumental undertaking. Numerous tools on the market let you experiment with ML and AI models in a sandbox, targeting small “problem areas” that might have long stymied your attempts at improvement. The important thing is that you need to get started soon, before the competition masters these tools and jumps ahead of you in the marketplace. While there is no silver bullet, it does help to get started now.
We at GroundHog can work with you to identify key areas where you can apply Machine Learning, collect relevant data, build and run machine learning models and then run a pilot to validate the base models that can be fine-tuned when you decide to apply it at a larger scale.
About GroundHog
easy to use connected miner software.
Get a 30 min. demo
Companies you know work with GroundHog
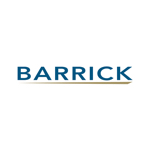
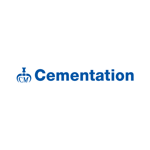
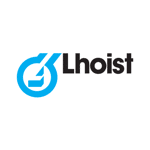
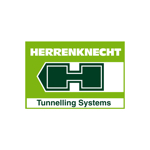
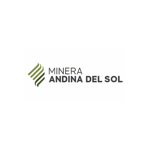
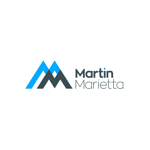
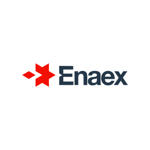
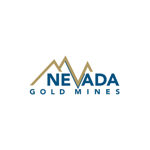